# Apps
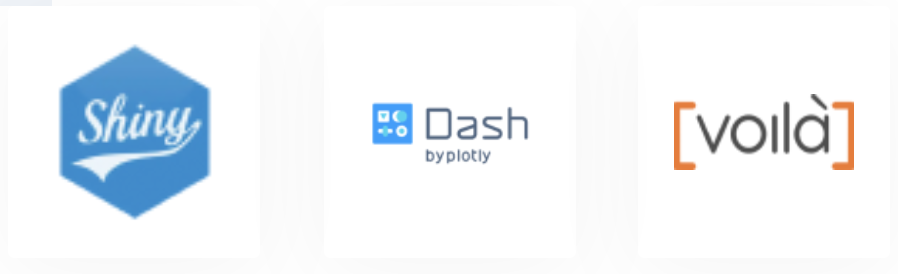
cnvrg is a full-stack platform, designed to simplify every aspect of your workflow.
One important part of a data scientist's work is presenting it to others. That project you’re working on won’t progress if you can’t effectively communicate your achievements. cnvrg simplifies this task.
cnvrg works easily and seamlessly with three major data communication platforms so you can use the application (app) most convenient and efficient for your and your team’s needs.
The topics in this page:
# R Shiny
Shiny is an R package that simplifies building interactive web apps straight from R. You can host standalone apps on a webpage, embed them in R markdown documents, or build dashboards. You can also extend your Shiny apps with CSS themes, htmlwidgets, and JavaScript actions.
# Use R Shiny
Complete the following steps to set up an R Shiny dashboard:
- Click Apps in your project.
- Select R Shiny.
- For File, enter the full path of the R Shiny
app.R
file. - In Advanced Settings, set the following image and compute:
- For Image, select the default
cnvrg_r
image. - For Compute, select the compute to use.
- For Image, select the default
- Click Publish. cnvrg accesses the compute and starts running the R Shiny server.
NOTE
The R Shiny file must be called app.R
.
If no path is given, app.R
is assumed to be in the project's parent directory. If you enter the full path (in the project) for File, for example, my_folder/app.R
, then that file loads from the correct subdirectory.
# R Shiny logs
The logs for the R Shiny server can be found within the compute at /var/log/shiny-server
.
# Customize the R Shiny configuration with a custom image
You can modify the R Shiny configuration to meet your requirements. By default, cnvrg starts the R Shiny server using the command exec shiny-server 2>&1
and runs using the following configuration:
# Instruct Shiny Server to run applications as the user "shiny"
run_as shiny;
# Define a server that listens on port 3838
server {
listen 3838;
# Define a location at the base URL
location / {
# Host the directory of Shiny Apps stored in this directory
site_dir /cnvrg; #or a path of the app.R file
# Log all Shiny output to files in this directory
log_dir /var/log/shiny-server;
# When a user visits the base URL rather than a particular application,
# an index of the applications available in this directory will be shown.
directory_index on;
}
}
If you want to start the server in a customized way, create a custom image and save your custom configuration file as /init. If /init exists, cnvrg uses it to start the Shiny server. Otherwise, it runs with the default server startup:
#!/bin/sh
# Make sure the directory for individual app logs exists
mkdir -p /var/log/shiny-server
chown shiny.shiny /var/log/shiny-server
if [ "$APPLICATION_LOGS_TO_STDOUT" != "false" ];
then
# push the "real" application logs to stdout with xtail in detached mode
exec xtail /var/log/shiny-server/ &
fi
# start shiny server
exec shiny-server 2>&1
# Dash
Dash is a productive Python framework for building web apps.
Written on top of Flask, Plotly.js, and React.js, Dash is ideal for building data visualization apps with highly custom user interfaces in pure Python. It's particularly suited for anyone who works with data in Python.
Through a few simple patterns, Dash abstracts away all of the technologies and protocols that are required to build an interactive web-based app. Dash is simple enough that you can bind a user interface around your Python code in a few hours.
Dash apps are rendered in the web browser. You can deploy your apps to servers and then share them through URLs. Since Dash apps are viewed in the web browser, Dash is inherently cross-platform and mobile-ready.
# Voilà
Voilà converts Jupyter notebooks into standalone web apps.
Not only does Voilà create visual graphs to make reporting easier, it also democratizes Jupyter notebooks for nontechnical users. With it, every notebook can be converted into a Voilà app. Better yet, you can create a customized dashboard with results of your machine learning project. Voilà supports Jupyter interactive widgets, including roundtrips to the kernel. It improves the conciseness by prohibiting arbitrary code execution by dashboard consumers.
Other benefits of Voilà are that it is built upon Jupyter standard protocols and file formats, and works with any Jupyter kernel (C++, Python, Julia). This makes Voilà a language-agnostic dashboard system. Additionally, extensible Voilà includes a flexible template system to produce rich application layouts. That means you can easily customize your dashboard based on the Jupyter notebook being deployed.
# App Deployment
Complete the following steps to deploy an app:
- Enter the project to publish your app from.
- Click Apps on the sidebar.
- Select the app type, enter the file to be used for the app, and allocate a compute.
- If necessary, select a dataset and complete the other options.
- Click Publish.
cnvrg completes the remaining required tasks.
# Deployed App Updates
You can update a deployed app without redeploying from scratch. Complete the following steps to update an app:
- Go to the app to update.
- Click Versions on the top menu bar.
- Select a new cnvrg.io Commit to update the service to.
- Click Update. cnvrg updates the app to use the newly chosen commit.
When connected to Git, clicking Update also pulls the latest Git commit for your branch (even if you do not choose a new cnvrg Commit).
← Serving Environments →